The ongoing deployment of IoT devices is rapidly changing the world. The subset of IoT known as Industrial Internet of Things (IIoT) is also making its presence felt, particularly in maintenance and asset management. This change is heralded both by the increasing number of IIoT devices in use and the growing popularity of digital twins. The upcoming launch of 5G wireless will likely see these devices become even more popular.
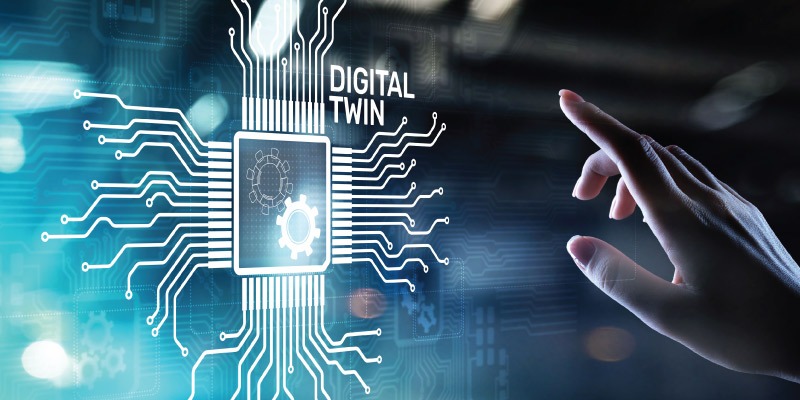
Digital twins are an increasingly common tool for maintenance professionals, allowing them to more fully reap the potential benefits of IIoT.
Before writing this post, I spent a lot of time trying to determine just how many IoT and IIoT devices are currently in use, but exact numbers have been difficult to pin down. Different studies performed by different organizations give different figures.
The research I’ve come across suggests a very conservative lower limit of around 7 billion devices in use as of 2018. Other studies have put forward the idea that there were about 10 billion in use at that time.
The lower limit given above means there are more IoT devices in operation than there are mobile phones. The high end of the estimates means there are fewer humans alive today than there are IoT devices. It seems certain that people are outnumbered by IoT devices by now, even if we take the lower limit of 7 billion in 2018 as gospel.
Let’s take a quick look at a few more stats before we dive into exactly how the growing IIoT universe is currently impacting maintenance management, the developments we might see in the near future, and some of the challenges adopters may face.
- A report from IDC forecasts that the IoT marketplace will be worth about $1.2 trillion by 2022.
- The same report shows that IoT in production asset management has the second-highest rate of investment at $44.2 billion, with only manufacturing operations investing more.
- A 2019 study by Gartner indicates that roughly three-quarters of organizations adopting IoT are also either adopting blockchain technology or intend to before the end of 2020.
- According to Ericcson, 3.5 billion cellular devices will include IoT connections by 2023.
- A study conducted in 2017 by Gartner indicates that 75% of organizations may not realize the full benefit of their own IoT technology, due to a lack of data science specialists.
Artificial Intelligence (AI) and Digital Twins
These are tremendously exciting times for maintenance, in no small part due to the increasing adoption of AI and predictive modeling through the use of digital twins. These are often very closely linked.
It should be noted that when we refer to “AI” in this context we are often talking about the subset of AI known as machine learning.
AI is a sort of umbrella term that refers to computers able to make decisions like a human being would: by weighing up the evidence, examining the current situation and its context, looking over the historical data, and then making a decision.
You can further divide AI into general and applied. Self-driving cars are one example of applied AI. They do that one task and nothing else.
A general AI, in theory, can do anything. It also doesn’t exist. We don’t insist that this will always be the case, but currently, there are no general AIs in existence. You may or may not see one in your lifetime.
Machine learning can be viewed as a type of applied AI: you give machines access to training data and the algorithm uses it to build models. It then uses these models to make decisions without being specifically programmed to do so.
The most obvious way this is transforming maintenance practices is in predictive analytics, a key component of any predictive or prescriptive maintenance strategy. The creation of automated analytical models can be developed from historical data and take into account both performance levels and external factors such as weather. This can be then compared against ideal performance data.
A sufficiently well-trained algorithm can then alert technicians that maintenance will be needed before a breakdown occurs, and even give a timeline for how soon the work must be completed.
Then we have the matter of inspections. This is a tedious and time-consuming activity. Even something as simple as reading and logging results uses up a lot of time that could be used for other tasks.
Sensors and IIoT solutions can help here in gathering data, and machine learning algorithms can be developed to interpret that data and give human technicians alerts when appropriate.
Digital twins, also known as cyber objects or digital avatars, are software versions of something that exists in the real world, such as a pump, conveyor belt, etc. In its simplest terms, it’s a digital copy of a physical asset. However, the process of using digital twins goes further than simply making a copy.
According to a study conducted by Gartner in 2019, 75% of organizations implementing IoT either already use digital twins, or plan to within a year.
A true digital twin of an asset involves the seamless transmission of performance and other data from the real-world asset to its digital copy. Once the digital twin has enough sensor data accrued from the physical asset, you can begin to model its future behavior with a high degree of accuracy.
This allows you to practice predictive maintenance, as you know with a great deal of certainty just when the physical asset will break down, what the cause of the failure is likely to be, and the best techniques for remediation. While the process itself carries a cost, it should be easy to recoup by making maintenance more effective and ensuring production is limited only for business reasons, not equipment failure.
Creating a Digital Twin
Building a digital twin requires an accurate, virtual model of the asset in question. This needs to be developed so it effectively captures all of the physical properties of the asset.
Data captured by the asset’s IIoT sensors is then fed into the digital model. The data includes information about the asset’s operating environment, current condition, and its performance.
The digital twin should also be integrated with your ERP system and any shop floor management systems to ensure it has access to operational data.
By analyzing incoming real-time sensor data and comparing it against historical data, failures can be predicted with increasing accuracy. With the right set-up, a digital twin can see that certain conditions are likely to lead to failure, notify the maintenance department, and even recommend what needs to be done to avoid downtime.
Learn more about Digital Twin Technology.