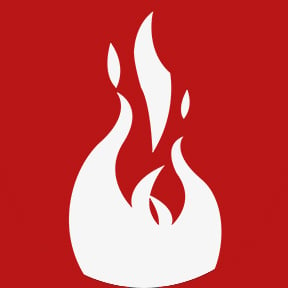
When Big Data is Too Big: Why Maintenance Needs a Robust Analytics Solution
Some of us struggle with too little data. However, it’s often the case in enterprise asset management that there’s too much data to leverage using traditional techniques. A robust analytics solution, designed for the needs of maintenance and asset management, is required to make effective use of the data. It’s not enough for the software to simply extract the data. The solution should provide various types of maintenance management reports that a team can use to make process and strategy improvements.
Roger Mougalas of O’Reilly Media first coined the term Big Data in 2005, but Big Data was already in existence before then. Mougalas was simply putting a name to a phenomenon that had already been observed. Organizations constantly generated mountains of data and could gain insights from this data if they had access to the right tools. Traditional management reporting software, however, wasn’t set up for this task.
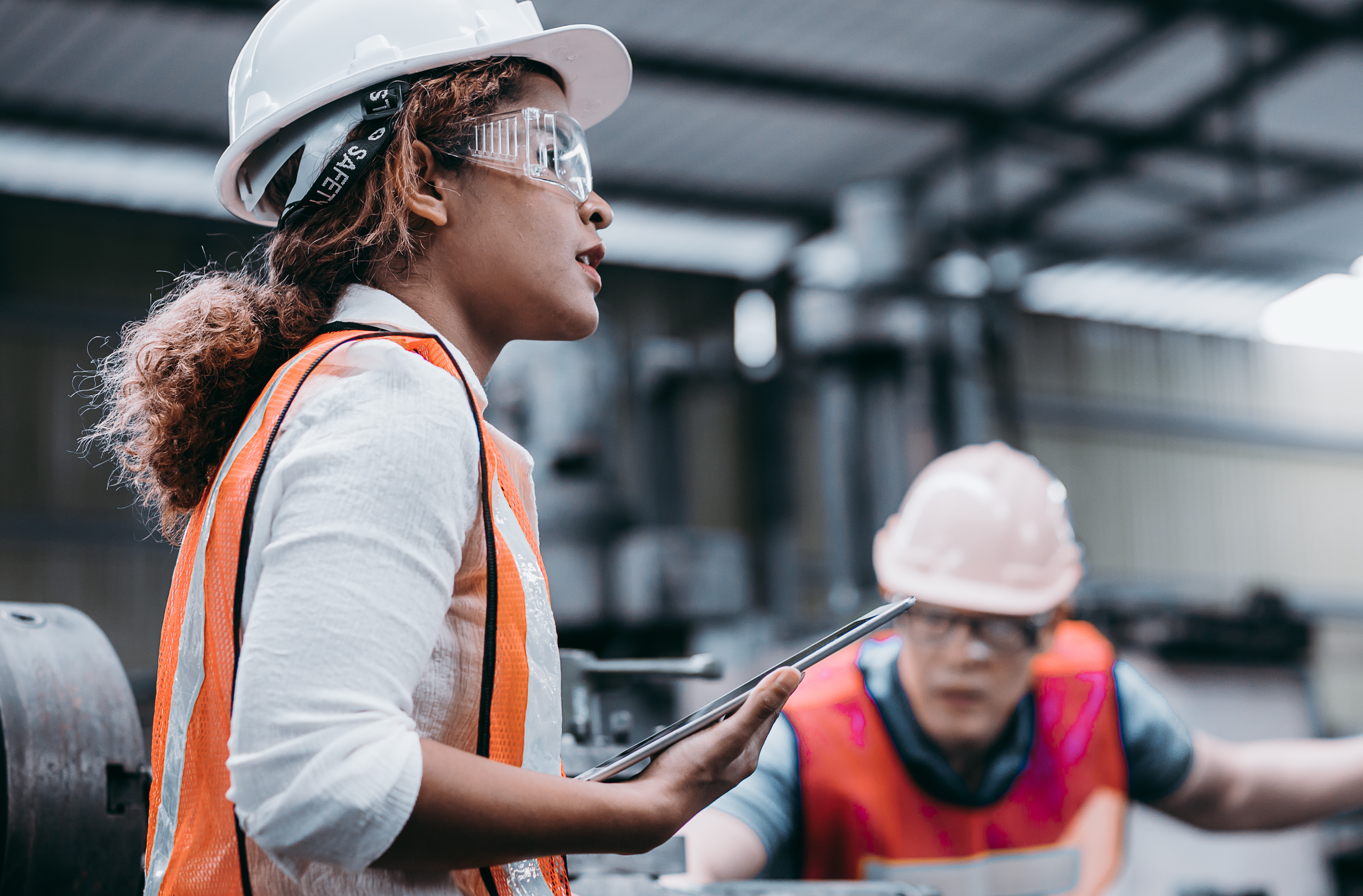
The essential characteristics of Big Data are defined by the three ‘Vs’: volume (there’s a lot of it), velocity (it’s coming in at high frequency, often in real time), and variety (both structured and unstructured data). Some definitions go further and add additional characteristics like veracity (the data must be true) and value (it must contain worthwhile insights). John Soldatos discusses these concepts in- depth in his blog, “Your Predictive Maintenance Capabilities will be Enhanced by Big Data Technologies.”
Large, high-quality data sets are a prerequisite for any Big Data project. Gathering enough data isn’t usually the problem in asset management. You may already have a wealth of data in your EAM, ERP, or CMMS, drawn from work orders, staff records, and asset history. The data may have to undergo an extensive cleansing to be useful, but it usually exists. Using Big Data in enterprise asset management often has two challenges:
1. Data is drawn without a clear business purpose in mind. You need to know what you’re looking for and why you’re looking for it. Without a clear goal in mind, you will simply drown the maintenance organization in data it doesn’t need and can’t use.
2. Too much data is presented, making it virtually impossible to draw useful insights. It’s not feasible for an organization to know what data to draw useful analysis from if there’s no organized way to manage the data. It can be compared to looking for a needle in a haystack, when all the hay is also made out of needles.
Many maintenance organizations are already drawing insights from this data, using traditional Business Intelligence (BI) tools. These often provide top-level yet highly valuable indicators on the relative success or failure of maintenance initiatives, such as schedule compliance. However, traditional BI tools don’t offer the granularity needed to make use of Big Data.
Your maintenance organization needs the insights that Big Data can provide. In turn, that means you need an analytics solution that is designed for enterprise asset management, with the ability to configure the maintenance management reports directly to your needs. Below we summarize a few of the most common challenges of trying to use traditional BI techniques on Big Data.
Alert Storms
You've got a wealth of data coming in. You might have data coming from sensors, while some is coming from physical inspections. An alert is created in the system every single time an anomaly is detected. The problem is that many of these alerts are simply showing you symptoms, rather than the root cause. All an alert shows is that something has deviated from the norm. Viewed in isolation, it cannot tell you if the incident that triggered the alert is a serious anomaly or a minor blip. Broadly speaking, the issue is one of false positives: alerts that look like a failure in the offing but turn out to be nothing.
Part of the trouble is that any given alert might indicate real danger to your assets. Discovering exactly what each alert means requires your team to dig into the notification and see what it truly indicates.
Automated anomaly detection is key to meeting this challenge. The right software solution can be configured so that important alerts are passed on, while lower-lever alerts are logged and become part of your historical data.
Threshold Creep
This is often related to the alert storm issue outlined above. A maintenance organization experiencing continual alert storms may decide that the best option is to simply change the thresholds for when alerts are created. For example, the default setting may be to create an alert if the temperature of an asset rises more than three degrees above its optimum operating temperature. However, it turns out that this happens multiple times per day. From the perspective of asset performance management, it doesn’t appear to have any impact at all.
There’s usually a bit of “cushion” built into recommendations, so you know you can probably raise that temperature threshold without negative repercussions.
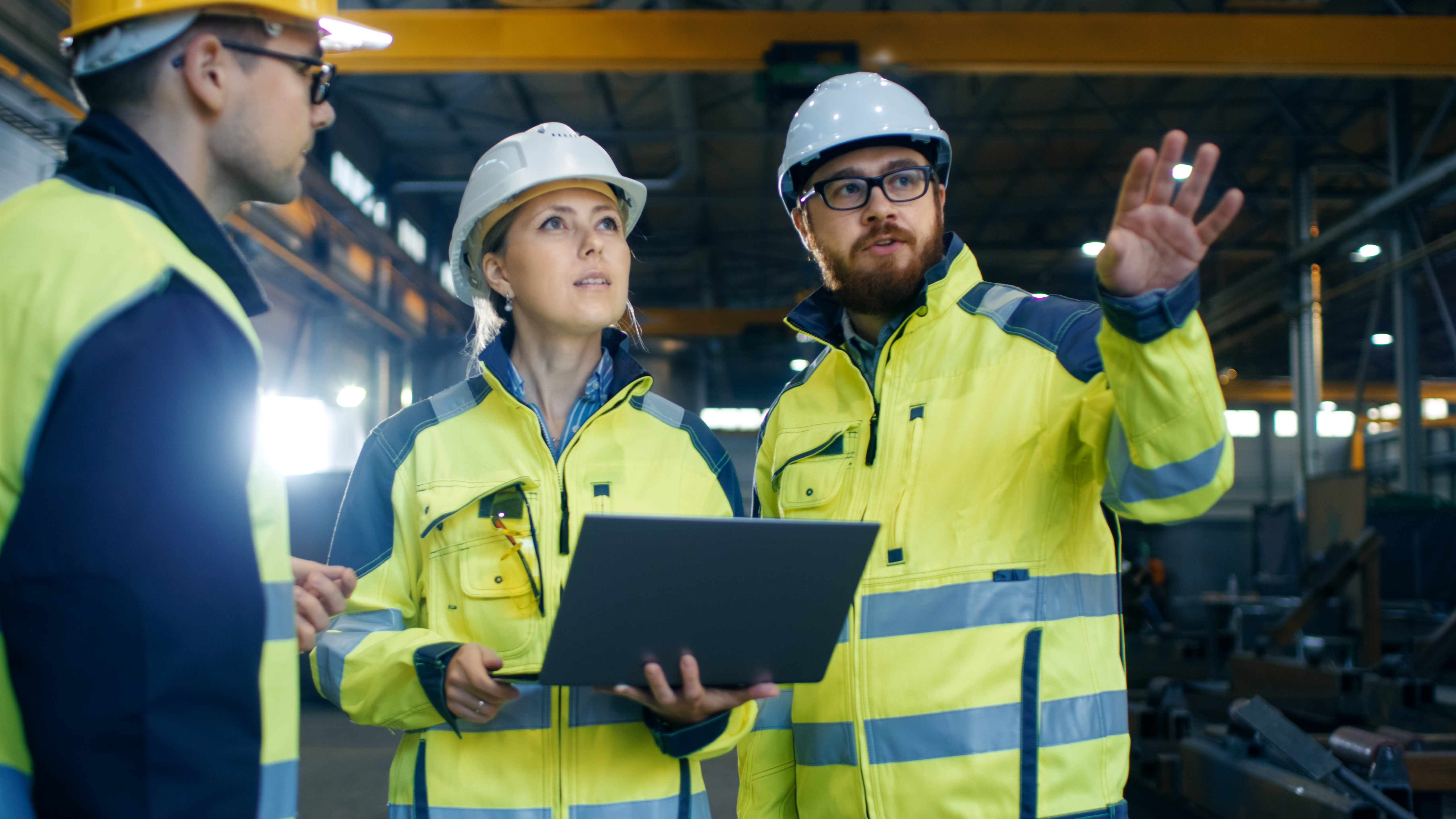
The temperature threshold is changed to four degrees to help cut down on the number of alerts. When this doesn’t solve the problem, the threshold is increased again, first to five degrees and later to six. Finally, the alert storms have stopped! Unfortunately, it turns out that you ran out of cushion at five degrees. The alert goes off at six degrees, but not in enough time to prevent the asset from failing.
This does not seem like a Big Data problem at first glance. However, it is directly caused by a lack of solutions suitable for parsing Big Data. The right software can be configured to only pass on alerts that have actual value, all without changing the thresholds for when events are logged.
Seasonality and Time Cycles
Most businesses are impacted by seasonality in one form or another. This seasonality can be challenging with Big Data if your software isn’t properly configured to account for these changes.
Data is constantly being gathered on your production activities. Traditional BI tools may very well treat an expected seasonal lull as an unexpected dip in production. Unless the system is configured to avoid this, it may trigger an alert storm. Traditional dashboards can’t keep up. Real alerts, ones that indicate a potential failure, may be missed.
The right analytics solution can help by excluding this seasonal data when necessary, while still keeping track of any critical alerts.
Prometheus Group solutions are designed with asset management and maintenance in mind. For more information on how Prometheus Reporting & Analytics can help you win with Big Data, reach out to us.