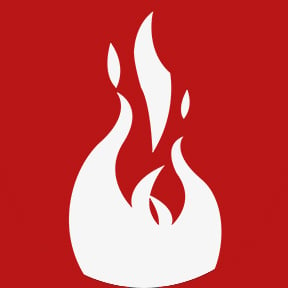
Why Maintenance Needs Master Data-as-a-Service
What is Master Data?
Master data management solutions have become a hot topic in the maintenance field over the last few years, but there is still some confusion about exactly what the term means. In the simplest definition, master data is consistently formatted and recorded data dealing with business objects: assets, employees, parts, vendors, and so on.
In short, master data is a set of common terms applied across the organization to define and describe business objects. One of the key distinctions is that master data deals with classifying and categorizing business objects, rather than transactions involving those objects.
Organizations are producing and compiling more data than ever before. With the explosion of Internet of Things (IoT) technologies and advanced digitalization initiatives, organizations are swimming in a sea of data and challenged to make sense of it all. Further complicating the data landscape is that data is scattered throughout the organization, often siloed in various systems of record and in countless formats. It is rarely in a single centrally managed location.
Making matters worse, different parts of the organization may use varying terms to describe the same thing. This conflict isn’t just limited to departments or business units. Often individuals within the same department use slightly, or in many cases, wildly differing terms to refer to the same items. This scattershot approach to master data management inevitably leads to inefficiency and ultimately hinders profitability.
The situation is confusing when one site refers to an electrical craftsperson as an “Elec. Craft” and another refers to someone with the same set of skills as an “Electrician.” The problem becomes much starker when we’re dealing with automatic data processing, such as in analytics or machine learning. These systems need terms that are clear, defined, and unmistakable.
What Isn’t Master Data?
-1.png)
There are some types of data that can be mistaken for master data if we’re not careful to use the right definitions. Below are some of the types of information that may be mistaken for master data.
Transactional Data: This describes events involving business objects rather than the objects themselves. This includes financial data, but work orders are probably the most common type of transactional data in maintenance. Your maintenance organization’s transactional data also includes invoices, inventory tracking, and so on.
Transactional data is usually distinct from master data, but there are rare occasions when an organization must use transactional data as master data. This occurs when an organization has master data entities that are only contained within transactional data. Contractors are one example in maintenance where the “master data” on those objects exists only as transactions.
Metadata: This is data about data. It can include a text description of the data, information on when the data was created, where and how the data is structured, and other aspects about the data itself.
Log Data: This type of data records events and processes that were used. Log data can be generated by physical inspections or remote sensors.
These types of data may revolve around business objects, but they do not classify or categorize them except under rare circumstances as noted above. This is what makes master data distinct.
The Traditional Approach to Master Data Management Solutions
Master data management (MDM) is the practice of ensuring our master data is maintained properly and used effectively. Before any of that, however, the master data itself must be created.
Create or otherwise acquire a taxonomy.
The taxonomy is a sort of “dictionary” used to define business objects (assets, locations, staff, etc.). Your taxonomy also lays out how your data is organized.
It’s impossible to overstate the importance of a robust and well-designed taxonomy. Whether it’s material, equipment, vendors, or staff, the taxonomy defines and organizes all of it.
Some organizations try to figure out how to build a taxonomy themselves, but a more common path is to work closely with subject matter experts who are specialists in master data management, and even more so, their industry. Building a taxonomy can be very challenging, even for experts. Just getting stakeholders to agree on the terms used can be an uphill battle.
Consolidate and cleanse the data.
This requires gathering all the data you have from diverse sources, such as your systems of record, engineering drawings, Excel spreadsheets, and so on. It can be cumbersome to make sure you’ve really gathered all the required data, but it’s relatively simple compared to the cleansing process.
-1.png)
Data cleansing consists of going through the data, line by line and record by record, and deleting bad data, removing duplicates, and building a master data set that is highly accurate, complete, and internally consistent.
This is a massive undertaking. These projects have historically been very long and require significant capital expenditure. Data cleansing is such a time intensive project that requires subject matter expertise, that it’s rare for an organization to complete it internally.
Bringing in experts reduces both time and costs associated with data cleansing projects. Master data projects are often undertaken alongside other projects, such as the implementation of a new ERP or major updates to a current system. Consultants help to bridge the time gap as well as supplying much needed expertise.
It may take a huge amount of time and money to get there, but once a taxonomy is in place and the data has been cleansed, the master data project will really start to demonstrate its business value. However, it will not retain that value without sustainment. This is one of the greatest challenges of the traditional approach to master data management.
Sustain the data.
Sustainment is the final stage of master data management, and it may be the trickiest one of all. Sustainment is the process of making sure that the data remains clean and any new data entering the system is standardized, follows definitive taxonomies, and applies best practices. Sustainment has no endpoint. It continues until the end of the organization or the end of time, whichever comes first.
Organizations are continually evolving and generating new master data, making sustainment a critical and ongoing component of any master data strategy. This occurs any time a new business object is added to the organization, including new assets and new hires. In addition, new data is continuously generated for existing business objects, and this data must be kept clean as well.
If everything goes perfectly, the data will stay clean. How often does everything go perfectly? Here’s a short list of potential ways that “dirty” data may be introduced into your system:
Experienced personnel may have trouble breaking their habits and accidentally use old formats
New personnel may not get the training they need (or may not have been paying attention)
The integration between systems may be spotty or nonexistent
Data governance and stewardship rules put in place may be insufficient to guard against incorrect or improperly formatted data
Most of these can be attributed to simple human error. It is technically true that every human error is avoidable, but there’s a reason they put erasers on pencils. It is unrealistic to expect everyone involved in a big, ongoing project to do exactly the right thing every single time.
These problems will stay with us as long as we are committed to a traditional approach to master data management. We can overcome these challenges, but it requires changing the way we go about creating, cleansing, and managing our master data.
What is Master Data-as-a-Service?
Prometheus Group launched Master Data-as-a-Service (MDaaS) in 2020 and it’s turned the entire concept of master data management on its head. Prometheus Group has extensive experience in master data consulting and cleansing with hundreds of enterprise customers across all asset intensive industries. This experience, and that of our customers—combined with that of our team of data scientists—was brought to bear in creating this new solution.
The MDaaS solution leverages this experience in several ways. First, it has led to the creation of a standard taxonomy that can be used by any industry.
“All of the pieces were there. Every company, every plant, has at least some common records, equipment, materials, and vendors,” notes Jeff Kinard, Senior VP of Strategic Partnerships at Prometheus Group. “Why can’t we just have one database that you offer to everyone with one standard?”
There are plenty of people who would be willing to say that this simply wouldn’t work. Every industry is different, and there are always significant differences between organizations within an industry.
This is true, but the long and varied experience of Prometheus Group’s consultants and customers made building a standard taxonomy possible. However, we recognized that this left the possibility of gaps. An additional layer of standards was added to the taxonomy to ensure it could handle literally anything.
“We’ve also leveraging other industry standards, like ISO 14224, PIDEX, and UNSPC codes to make it as complete as possible,” says Jeff.
Since its initial creation, the MDaaS taxonomy has only increased in size and power. It started as a best-in-class industry standard, and currently it includes a noun modifier dictionary for materials, a nameplate dictionary for equipment, catalogue profiles with industry standard object parts, as well as a complete library of damage, cause, and activity codes.
The colossal amount of data gathered for the MDaaS project has also provided an opportunity for Prometheus Group to examine best practices and use them to improve parts of the service. MDaaS now features optimized maintenance plans and tasks lists, standardized measurement points and value thresholds, and other features.
MDaaS employs an industry leading machine learning engine fueled by two decades of master data experience and the industry’s largest proprietary asset and materials repository to automate data cleansing and enrichment.
-1.png)
MDaaS eliminates all the traditional challenges associated with master data management, from building a taxonomy through to sustainment. This solution not only beats the traditional approach, but it’s also often less expensive as well. Instead of high capital expenditures for cleansing and sustainment, organizations can pay a relatively small fee as an operating expenditure.
The traditional approach to master data only treats symptoms. Without continuous, high levels of investment, a lack of sustainment means those symptoms are always lurking beneath the surface. MDaaS is a revolutionary solution that treats the illness and eliminates it.
For more information on MDaaS, please see our Master Data page or contact us here.